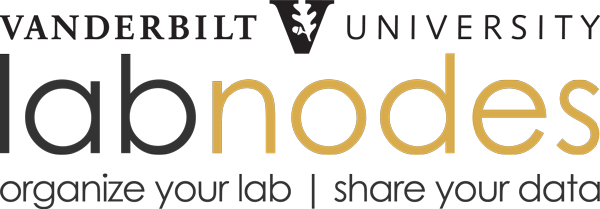
Thank you and farewell!
Labnodes was launched in response to the refreshing of the Basic Science Strategic Plan for the Vanderbilt University Medical Center, which took place in early 2008. At that time, VUMC investigators expressed the need for a web-based platform that would enhance communications, facilitate collaborative research and streamline regulatory compliance.
Labnodes provided a platform for "connecting the dots" between people, communities, resources and publications, and did so via intuitive, dashboard-type views. Over nearly a decade, 2,500 registered users made use of Labnodes to build nearly 2,800 communities/workspaces, to enter descriptions of 17,000 resources, and annotate over 40,000 publications.
In October of 2022, the server that Labnodes ran on was compromised, forcing us to take Labnodes offline, at least for now. Data within Labnodes can still be accessed by special request. If there is something you need, please let us know.
For those who used and supported Labnodes, we thank you!
JP Cartailler & Mark Magnuson
Vanderbilt Center for Stem Cell Biology & Creative Data Solutions